Divergent in the workplace: Maggie Cheng looks at what pop culture can teach us about hiring
Contributed by Izabela Szydlo, DeGroote Research Writer
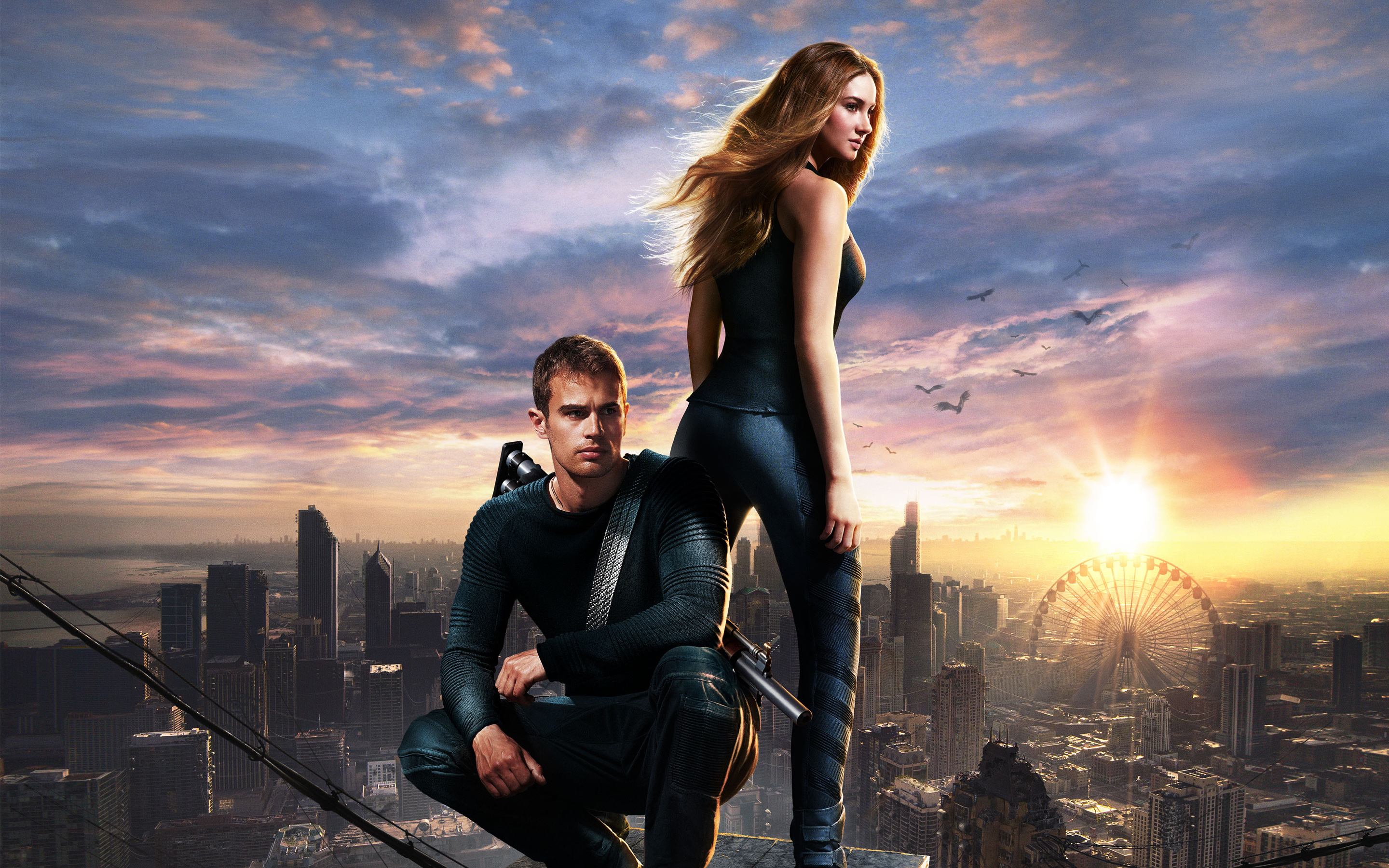
Theo James and Shailene Woodley star in the Divergent film series. “By building an analytical system to manage employees, are we attempting to differentiate them based on stereotypes so that we can control them?” asks DeGroote PhD candidate Maggie Cheng.
Veronica Roth’s Divergent is the story of teenagers living in a dystopian world, where they are divided into factions based on skills and attributes. Teens who possess traits of multiple factions, meanwhile, are called Divergent. They have independent thoughts and are considered threats.
For Maggie Cheng, a DeGroote PhD candidate in Human Resources and Management, Divergent helped inspire an award-winning paper that examines potential negative implications of using analytics to manage human performance and predict behaviours.
Cheng’s paper, Causal Modeling in HR Analytics: A Practical Guide to Models, Pitfalls, and Suggestions, recently won the Information Age Publishing Outstanding Doctoral Student Paper on Management Consulting award at the Academy of Management Annual Conference. The paper also garnered the Management Consulting Division Best Paper Award.
“My starting point was quite simple: By building an analytical system to manage employees, are we attempting to differentiate them based on stereotypes so that we can control them?” Cheng asks. “Can we build a system that could correct the existing biases and discrimination in workplaces, and truly focus on the well-being and advancement of humans?”
Using Roth’s story as an analogy, employees should strive to be Divergent, Cheng explains. They should be encouraged to think critically, offer constructive feedback, and seize developmental opportunities. We need to be aware of the limitations of analytics that could hold us back from achieving this goal, she adds.
Causal modeling, the foundation of her paper, involves the use of statistics to determine which factors actually affect a given outcome. A classic example is the high correlation between ice cream sales and crime — when ice cream sales are high, so is crime. If just the correlation (absent casual modeling) was trusted, one might assume that preventing the sale of ice cream would result in less crime. However, the real culprit is the increase in temperature, which accounts for increases in both ice cream sales and crime.
“We generalize things based on our observation of two things occurring at the same time, and that’s where the biases come from,” says Cheng. “If we build human resources analytics software packages on the same logic, that could result in a similar deviation from truth.”
Like the ice cream example, if human resources analytics are purely based on data correlation, potentially harmful conclusions could be drawn. For example, using non-causal analytics in staffing may not only lead to poor hiring decisions, but could result in human rights violations, Cheng says.
“Statistically speaking, minorities often fit into certain stereotypes,” she says. “However, using these stereotypes, along with correlated results and coincidences, can lead to awful consequences.” Avoiding certain candidates will not produce a smarter or better workforce, Cheng warns. Instead, such practices could eliminate qualified candidates while ultimately leaving an organization open to legal action.
Maggie (Minghui) Cheng joined DeGroote’s PhD program in September 2013. She had previously worked in the national news agency of China with extensive experience in news translating, reporting, and staff management. Her research interest lies in ethics, leadership, gender and organization, and data analytics in human resources management.
Comment Policy
We generally welcome discussion on our blog posts. However, we reserve the right to edit or delete comments in certain situations:
This site is moderated by the DeGroote Marketing and Communications team. If you have any questions please email Katie Almas.